Economic Decline Over Three Decades: The article highlights the Bureau...
Read MoreLatest News
Rand Faces Potential Depreciation to R21.40 Against Dollar
Investec's Warning: Investec, a leading financial institution, issues a cautionary...
Read MoreEskom’s Load-Shedding Woes Linger Despite Recent Relief
Despite a recent stretch of over 28 days without load-shedding,...
Read MoreCapitec CEO’s Whopping R65 Million Payday Raises Eyebrows
Financial Performance: Capitec Bank reports strong growth in headline earnings...
Read MoreEditor's Pick
Ripple’s Dip Amid SEC Clash Opens Buy Opportunity for XRP
Ripple (XRP) price falls to $0.5623 (R10.49), influenced by a...
Read MoreOndo’s Price Eyes $1 Milestone with Key Support Retake
Ondo (ONDO) is showing potential for recovery, closely following broader...
Read MoreNear Protocol’s Price Surge: A Potential Windfall for Investors
The Near Protocol (NEAR) cryptocurrency has overcome two major resistance...
Read MoreBitcoin Faces Hurdles: R1.3M Key for Pre-Halving Price Surge
Bitcoin's price struggles to surpass the $69,000 (approximately R1,300,650) mark,...
Read MorePopular Posts
How to reverse Capitec Bank cash send
The Capitec Bank Cash Send has become an alternative method...
Read MoreSouth African Police Service (SAPS) Salary Guide 2024
In 2024, the compensation landscape for police officers in South...
Read MoreSouth African Driver’s Licence Renewal Guide 2024
In South Africa, a driver’s license has a 5-year lifespan;...
Read MoreFNB Black Card 2024 Review
The FNB Black Card series, tailored for high-net-worth individuals and...
Read MoreEnergy
- Eskom’s Load-Shedding Woes Linger Despite Recent Relief
- South Africa’s Energy Outlook Brightens as Renewables Gain Momentum
- Charlotte Maxeke Hospital Grapples with Ongoing Power Outages
- Illegal Electricity Connections Claim Three Lives in Gqeberha Tragedy
- Eskom Achieves Milestone: Rolling Blackouts Suspended Indefinitely
- Power Crisis: Eskom Battles Major Outage in Pretoria
- South Africa’s Path to Energy and Water Resilience
- Eskom’s Electricity Prices Soar with New Tariffs
- Eskom’s Load Shedding Pause & Tariff Hike Explained
- City Power Cracks Down on Electricity Theft With Smart Meters
Business
- ABSA Bank: AMB285 Redemption Alert
- Akani 2 Invests Big: Acquires 20.43% Stake in WBHO
- PSV Holdings Navigates Complex Financial Challenges, Stakeholder Dynamics, and Legal Battles
- Pravin Gordhan Assures SAA’s Survival and Growth Plans
- A Deep Dive into SPAR Group’s Voluntary Trading Update
- YeboYethu Announces FY 2023 Results and Dividend Declaration
- Orion Minerals Announces Board Changes Amid Transition to Development Stage
- Discovery Limited: Two New Directors Boost Global Leadership
- WeBuyCars Reports Strong Growth Amid Plans for Separate Listing
- Libstar Holdings Limited: Analyzing Financial Performance and Strategic Initiatives
Tax
- Revolutionizing Tax Compliance: The Power of Collaborative Bargaining
- SARS Achieves Historic Tax Revenue Milestone
- SARS Boosts Tax Process: Smoother 2023 Changes Ahead
- Mobile Tax Unit Rolls into Limpopo: Get Hassle-Free Tax Services at Your Doorstep!”
- SARS Admin Penalties: Navigating the Tax Maze for South African Residents and Expats
- Revolutionizing Tax Returns: SARS Unveils Major Updates to Corporate Income Tax System
- SARS Advocates for eFiling: A New Era of Efficiency for Tax Practitioners
- SARS Elevates User Experience: Major eFiling Upgrade Scheduled for Enhanced Security and Efficiency
Technology
- OpenAI Dismisses Elon Musk’s Lawsuit, Claims Minimal Impact on Development
- WhatsApp Introduces “Search by Date” Feature for Android Users
- South Africa on Alert: TikTok’s Global Bans Raise Security Concerns
- Brilliant Labs Unveils Frame: Lightweight AR Glasses with AI Assistant Noa
- Asus Unveils Impressive OLED Monitors with Sky-High Refresh Rates at CES 2024
- Netflix’s DVD Delight: Free Discs, Extended Returns Enrich Experience
- Sony Unveils Project Q: The Ultimate Handheld for Streaming PS5 Games
- Brave Launches AI-Powered Assistant, Leo, for Android Users
- iPhone 15: USB-C & Thunderbolt Bring Desktop Revolution
- 8BitDo Unveils Micro: Ultimate Pocket Gaming Power for Nintendo & Android
- Meta Drops Messenger Lite, Shifts Focus to Main App
- Beats Unveils Studio Pro: Enhanced Sound and Versatility at ZAR 6,481
- Microsoft Teams Unveils Immersive 3D Meetings with Microsoft Mesh Integration
- Apple Card Users Earned Over $1 Billion in Rewards in 2023
- Snapchat’s My AI: Glitchy Story Sparks Buzz and Speculation
Crypto
- Pepe Coin Leaps Ahead: Meme Coin Market Sees Exciting Gains
- Ethereum Navigates Uncertain Waters, Eyes Potential ETF-Driven Price Surge
- USDC is becoming a more popular stablecoin than USDT
- Aptos Eyes Major Gain Amid Market Watch: What’s Next?
- Goldman Sachs Clients Embrace Bitcoin ETFs, Spark Crypto Surge
- Ethereum Staking Hits Peak; Price Rebound Hints at Gains
- Crypto.com (CRO) falls 30% as staking rewards are reduced
- Bitcoin Nears R1.5 Million Mark, Outpaces Gold in ETFs
- XRP Plummets, Traders Face R471m Loss Amid SEC Suit”
- Ethereum’s Future Hangs in Balance Amid US Congress Inquiry
- MakerDAO’s ‘Endgame’ Plan Aims to Revolutionize DeFi, Targets $100B
- China returns as 2nd largest Bitcoin mining hub despite the ban on crypto
# | Name | Price | Changes 24H | Available Supply |
---|
Careers
- Average Dental Hygienist Salary in South Africa 2024
- 3 Tips to Stay Employable During Times of Uncertainty
- Average Farm Worker salary in South Africa 2024
- Average Physiotherapist salary in South Africa 2024
- The Entrepreneurial Dream: The Benefits of Owning Your Own Business in South Africa
- Average Business Analyst salary in South Africa 2024
- Average Managing Director salary in South Africa 2024
- 20 Jobs to be Replaced by ChatGPT in South Africa: The AI Revolution Transforming the Job Market
- Average Waiter/Waitress salary in South Africa 2024
- Average Proofreader salary in South Africa 2022
Government
- South Africa’s EPWP Marks 20 Years with 5 Million Jobs
- President Ramaphosa Mourns Passing of Former Canadian PM Mulroney
- Public Protector Enquiry Progresses: Committee Submits Written Questions on Fitness to Hold Office
- South Africa: Corruption Scandals, Municipal Crackdowns, and Market Updates
- RAF Frauds Threaten Accident Victims’ Rights
- Potchefstroom Unrest Sparks Calls for Municipal Split Ahead of Elections
- Family’s Fight: Justice for Assaulted 13-Year-Old Victim
- Rising Petrol Station Hijackings Spark Urgent Motorist Concerns
- Fatal Shoot-Out: Three Suspects Killed in Johannesburg Confrontation
- SA GHS 2022: Household Trends, Vax Gaps, Service Advances
Economics
- FF+ Warns: Land Expropriation Threatens South Africa’s Economy
- Eastern Cape’s N2 Project Generates 3,000+ Local Jobs
- South Africa’s Black Industrialists Shine in Economic Empowerment Summit
- 5 best quick loans for emergency cash in 2024
- President Ramaphosa Urges Action on South Africa’s Education Crisis
- Johannesburg Water Crisis: Residents Protest as Suburbs Endure Drought
- Average General Worker Salary in South Africa 2024
- South African Businesses Embrace Innovation for Global Competitiveness
- South Africa’s Economy Shows Marginal Growth Amidst Sectoral Challenges
- South African Ministers Establish Task Team to Combat River Pollution
Personal Loan Calculator
You can never have financial freedom if you borrow without calculating. You need to know how much you will pay, understand if you can afford the loan.
Latest Financial Updates
We’re with you through thick and thin, updating you every step of the way. Our dedication is to helping you save money, and attain financial freedom.
Reviews
We are always looking for better financial deals for you, culminating to insightful reviews.
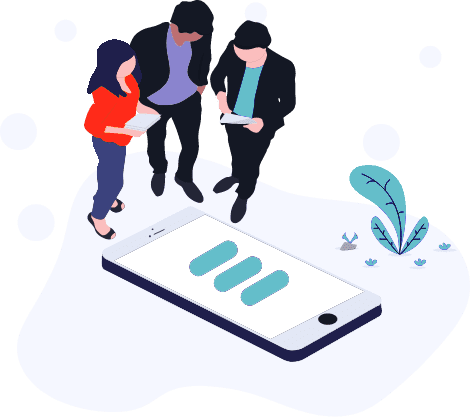
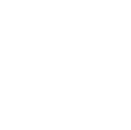
Investment Tips
There can be no Financial Freedom without sound investments.
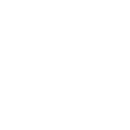
Business Resources
Find latest financial resources for your business. From loans to service providers.
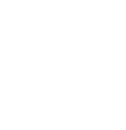
Career Tips
We share career tip to help you move from one level of financial freedom to another
Disclaimer
Rateweb strives to keep its information accurate and up to date. This information may be different than what you see when you visit a financial institution, service provider or specific product’s site. All financial products, shopping products and services are presented without warranty. When evaluating offers, please review the financial institution’s Terms and Conditions.
Rateweb is not a financial service provider and should in no way be seen as one. In compiling the articles for our website due caution was exercised in an attempt to gather information from reliable and accurate sources. The articles are of a general nature and do not purport to offer specialised and or personalised financial or investment advice. Neither the author, nor the publisher, will accept any responsibility for losses, omissions, errors, fortunes or misfortunes that may be suffered by any person that acts or refrains from acting as a result of these articles.